SASA 20216 Workshop 1
Computational Developments in High Dimensional Models
Monday 28 November, 9am to 4pm
This workshop will focus on both theoretical and computational developments in high-dimensional statistical models. Of particular interest are models that involve high-dimensional vector or matrix parameter estimation, such as those occurring in copula models, graphical and network models, factor models, and functional data. These models usually depend on parameters with sparse patterns. High-dimensional models with low-dimensional structure are crucial for the successful implementation and theoretical analysis of such models, especially for a limited amount of data. Rich applications occur in genetics, neuroscience, economics, public health, psychology and sociology. New scientific challenges in these established areas, or in other emerging areas arise on a continual basis.
The one day workshop on High Dimensional Statistics will include lectures by internationally prominent researchers, chosen by the organising committee and will cover many aspects of this theme, motivated by many applications. The aim of the workshop is to bring together researchers and scientists interested in high dimensional statistics, its applications, and related areas. In the afternoon there will be lectures and a computer lab session on longitudinal and functional data analysis.
Workshop organiser
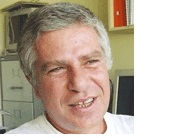
Professor Anestis Antoniadis
Laboratoire Jean Kuntzmann
University Joseph Fourier, Grenoble
&
Department of Statistical Sciences
University of Cape Town
Short CV
Anestis Antoniadis is an emeritus professor at the Joseph Fourier University in Grenoble and an honorary research associate at the University of Cape Town. His research interests include abstract inference for stochastic processes, nonlinear regression, nonparametric curve estimation, survival analysis and point processes, wavelets and their applications. On the applied side, he has worked on meteorology, climatology, crystallography, quality control, petrol products, air-pollution studies and micro-array data. He is a fellow of the Institute of Mathematics and the American Statistical Association, an elected member of the International Statistical Institute, and honorary member of the French Statistical Society. He was the Laplace Lecturer in the 8th World Congress in Probability and Statistics in 2012. He has been editor or associate editor of numerous journals.
Invited speakers
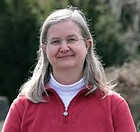
Professor Irène Gijbels
Department of Mathematics and Leuven Statistics Research Centre (LStat)
KU Leuven
Celestijnenlaan 200B, Box 2400, B-3001 Leuven (Heverlee), Belgium
Quantile regression in varying coefficient models
Quantile regression is an important tool for describing the characteristics of conditional distributions. To allow for analyzing complex data situations, several flexible regression models have been introduced. Among these are the varying coefficient models, that differ from a classical linear regression model by the fact that the regression coefficients are no longer constant but functions that vary with the value taken by another variable, such as for example time. In this talk we study quantile regression in varying coefficient models for longitudinal data. The quantile function is modeled as a function of the covariates and the main task is to estimate the unknown regression coefficient functions. We approximate each coefficient function by means of P-splines. Theoretical properties of the estimators, such as rate of convergence and an asymptotic distribution are established. We further consider several other aspects of the problem studied. Firstly, we pay attention to the property that population conditional quantile functions cannot cross for different quantile orders, and hence ensure that the estimated quantile curves do not cross. Secondly, we
allow for some heteroscedasticity in the error modelling, and also estimate the associated variability function. Finally, we briefly discuss other interesting problems that have been addressed, such as testing for a specific variability structure, or constrained inference for the varying coefficients. Some illustrations of the developed methodology with simulated data and real data analysis are provided.
This talk is mainly based on joint work with Yudhie Andriyana and Anneleen Verhasselt.
Short CV
Irène Gijbels obtained a PhD in Sciences (Mathematical Statistics) in Belgium, and after some postdoctoral stays at the University of North Carolina at Chapel Hill, USA, (with a Fullbright Hays scholarship) and the Mathematical Sciences and Research Institute in Berkeley, she was affiliated with the Université Catholique de Louvain. Since 2004 she is Full Professor at the University of Leuven (KU Leuven), Belgium, and heads a research group in Mathematical Statistics at the Department of Mathematics.
She is a Fellow of the IMS, the ASA and Elected member of the ISI. She was the previous Editor-in-Chief of Journal of Nonparametric Statistics, and continues to serve on editorial boards of major statistics journals. In addition, she provides services to several national and international scientific committees.
She has a large track record of publications in international peer-review journals and is co-author of the book on "Local Polynomial Fitting and Its applications", published with Chapman and Hall.
She recently was elected a member of the Royal Flemish Academy of Sciences.
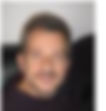
Professor Jean-Michel Poggi
Laboratoire de Mathématiques d’Orsay, Univ. Paris-Sud, Orsay, France
and
Université Paris Descartes, Paris, France
Random Forest-Based Approach for Physiological Functional Variable Selection for Driver's Stress Level Classification
With the increasing urbanization and technological advances, urban driving is bound to be a complex task that requires higher levels of alertness. Thus, the drivers mental workload should be optimal in order to manage critical situations in such challenging driving conditions. Past studies relied on drivers performances used subjective measures. The new wearable and non-intrusive sensor technology, is not only providing real-time physiological monitoring, but also is enriching the tools for human affective and cognitive states monitoring.
This study focuses on a drivers physiological changes using portable sensors in different urban routes. Specifically, the Electrodermal Activity (EDA) measured on two different locations: hand and foot, Electromyogram (EMG), Heart Rate (HR) and Respiration (RESP) of ten driving experiments in three types of routes are considered: rest area, city, and highway driving issued from physiological database, labelled "drivedb", available online on the PHYSIONET website.
Several studies have been done on driver's stress level recognition using physiological signals. Classically, researchers extract expert-based features from physiological signals and select the most relevant features in stress level recognition. This work aims to provide a random forest-based method for the selection of physiological functional variables in order to classify the stress level during real-world driving experience. The contribution of this study is twofold: on the methodological side, it considers physiological signals as functional variables and offers a procedure of data processing and variable selection. On the applied side, the proposed method provides a "blind" procedure of driver's stress level classification that do not depend on the expert-based studies of physiological signals.
This talk is mainly based on joint work with Neska El-Haouij, Raja Ghozi, Sylvie Sevestre Ghalila and Méeriem Jaidane.
Short CV
Jean-Michel Poggi is Professor of Statistics at Paris-Descartes University and Lab. Maths Orsay University. His research interests are in time series, wavelets, tree-based and resampling methods, as well as applied statistics. Research activities combine theoretical and practical contributions together with industrial applications (mainly environment and energy) and software development.
He is Elected Member of the ISI. He is Associate Editor of three journals: Journal of Statistical Software, CSBIGS (Case Studies in Business, Industry and Government Statistics) and Journal de la SFdS. From 2011 to 2013, he was President of the French Statistical Society (SFdS). He is President of ECAS, Vice-President of FENStatS, Vice-President of ENBIS. He is member of the Board of Directors of the ERS of IASC and Member of the EMS Committee for Applied Mathematics.
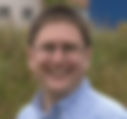
Professor Idris Eckley
Mathematics and Statistics, Lancaster University, Lancaster, LA1 4YF
Contemporary challenges in multivariate change-point analysis
In recent years there has been a surge of interest in the development of new methods for tackling change-point problems. Much of this work has focused on the analysis of univariate time series, with contributions in this area having an impact in various disciplines, notably climate science and genomics. However with the increased use of low-cost sensors, there is a growing need to be able to effciently and accurately identify changes in structure within highly multivariate time series. In moving to this more complex setting, the problem of detecting change-points becomes more subtle. We will outline some recent developments in this area motivated by ongoing work with industrial collaborators.
Short CV
Idris Eckley joined the Statistics Group at Lancaster University in August 2007. Prior to starting at Lancaster University, he worked as an internal statistical consultant at Shell Global Solutions.
At Lancaster he leads the Change-point and Non-stationary Time Series research group. He is also Co-Director of the EPSRC-funded STOR-i Centre for Doctoral Training. Together with Jon Tawn and Kevin Glazebrook, he led the development of STOR-i in 2010, which was subsequently renewed in 2014 (until 2022). Within STOR-i he leads the Centre's industrially-engaged research activity.
His current Interests lie in the development of change-point methods (including the development of change-point search algorithms) and multi-scale methods for the analysis of non-stationary time series. Other areas of interest include statistical approaches to texture analysis; the development of novel classification methods such as boosting methods and approaches for dealing with streaming data; the application of statistics within Business and Industry.
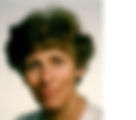
Professor Marie Hušková
Charles University in Prague
Department of Probability and Mathematical Statistics
Division of Mathematical Statistics
Sokolovská 83, 186 75 Praha, Tschechische Republik
Change point detection in panel data
The talk presents recent results on testing and estimating for a change in panel data. Asymptotic properties of the suggested procedures are presented. It is assumed that either the number of panels is large and the number of the observations in each panel are large or fixed (small w.r.t. the number of panels). Results of a simulation study and an application to a real data set will be presented.
This talk is based on joint work with several co-authors.
Short CV
Marie Hušková has been working in the Department of Probability and Mathematical Statistics of the Charles University in Prague since 1964 and got her professorship there in 1997. She is interested in rank statistics, adaptation, robustness, and change-point problems. She is the author of more than 130 scientific papers. She has an outstanding service as an editor and organizer of several International conferences. She is a member of the ISI, the Bernoulli Society, American Statistical Association and a fellow of the Institute of Mathematical Statistics.