SASA 20216 Workshop 4
STATISTICAL LEARNING IN DATA SCIENCE
Monday 28 November, 12:30pm to 4:30pm
We give an overview of statistical models used by data scientists for prediction and inference. With the rapid developments in internet technology, genomics, financial risk modelling, and other high-tech industries, we rely increasingly more on data analysis and statistical models to exploit the vast amounts of data at our fingertips. We then focus on two important classes of tools. For wide data, we have a closer look at the lasso and its relatives, and for tall data random forests and boosting.
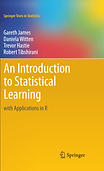
Most of the material can be found in “An Introduction to Statistical Learning, with Applications in R” by Gareth James, Daniela Witten, Trevor Hastie and Robert Tibshirani (Springer, 2013), which is also available free in pdf format from
http://web.stanford.edu/~hastie/local.ftp/Springer/ISLR_print6.pdf.
Presenter

Professor Trevor Hastie
The John A. Overdeck Professor
Professor of Statistics
Professor of Biomedical Data Science
Stanford University
Trevor Hastie has been a professor in the Statistics and Biostatistics Departments since 1994. He is founder and co-director of the Statistics department's industrial affiliates program. He has coauthored six books, among them the best-selling "Elements of Statistical Learning". He was awarded the Technometrics Ziegel award (dated 2014) for his book “An Introduction to Statistical Learning”, as well as the 2014 Parzen prize for statistical innovation.
His research interests include semi-parametric regression models, computer intensive data analysis techniques, statistical computing and consulting. He is currently working on adaptive modelling and prediction procedures, signal and image modelling, and problems in bioinformatics with many more variables than observations. He is an elected fellow/member of SASA, RSS, ASA, IMS and ISI.